
Advice
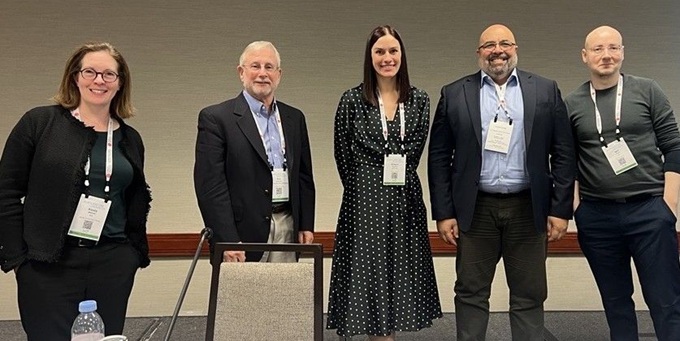
LLMs to Agentic AI - Tackling Trust and Explainability
Legalweek 2025 Session Recap
What does it mean to trust AI? According to AI expert Ron Brachman, “it’s when technology demonstrates consistent behaviour over time.”
Trust is a cornerstone of any successful AI deployment. Without trust, users and stakeholders are unlikely to embrace AI technologies, regardless of their current and future benefits.
As AI continues to advance, particularly with the advent of large language models (LLMs) and Agentic AI, trust remains top of mind and was the focus of the Legalweek 2025 Emerging Tech session presented by Epiq, “LLMs to Agentic AI — Tackling Trust and Explainability.” Moderated by Alexis Mitchell, Director, Advanced Technologies at Epiq, the session featured the following panellists:
- Ron Brachman, Visiting Professor, Cornell University, and Distinguished Visiting Scientist, Schmidt Sciences
- Allison Kostecka, Partner at Gibson Dunn
- Stephen Dooley, Director of Electronic Discovery and Litigation Support, Sullivan & Cromwell
- Igor Labutov, Vice President, Epiq AI Labs, Epiq
Moving Beyond Hallucinations
LLMs provide believable answers, however, they can also produce results that look plausible, but which can contain subtle errors, often referred to as ‘hallucinations.’ This was demonstrated in the session by Igor Labutov when he asked an LLM to write his bio, and the system produced believable affiliations that were totally made up. While the term hallucination is typically associated with LLMs, these types of confabulation errors are inherent in any machine learning model, and legal professionals have become familiar with them when working with TAR or other first-generation review technologies. As Labutov stated, “the challenge now is to develop confidence and trust in workflows that deal with more complex models capable of providing narrative responses.”
The discussion then shifted to recent AI advancements, reasoning models, and Agentic AI. Reasoning models can reflect on their outputs, reducing the chance of errors, but still have limitations in ingesting large document collections. Agentic AI mimics human workflows by using tools to take actions and feedback to refine answers.
It was emphasised that Agentic AI is a broad term, and an AI agent is not monolithic, but rather a complex system engineered by a solution provider — the majority of the system is not the AI part, but rather, scaffolding and connecting code that allows the agent to interact with other software systems. This architecture offers greater flexibility to address the limitations of LLMs. However, it also makes Agentic AI solutions difficult to compare without a clear understanding of their internal design, which is essential for proper evaluation. When choosing between different agent systems, Labutov noted that, “although there are many, many, different models, they’re all fundamentally very similar in their architectures.”
The Most Common Trust Challenges
As the legal industry looks to embrace advanced AI and build trust in using it, there is a need for industry-wide benchmarks and collaboration to establish standards for evaluating AI tools. The panel agreed that some of the most common ‘trust challenges’ the industry faces today include ethical and professional standards, explainability and transparency, accuracy and reliability, and practical operational factors. Stephen Dooley emphasised that explainability and transparency are crucial for defending not only the solution but also the workflow. He stressed the importance of having a historical and tracking solution to manage how tools handle data. Further, he highlighted the need for quality control checks to ensure the accuracy and reliability of AI models.
Another challenge concerns the users of advanced AI and the potential of putting too much trust in the answers they are generating. As AI continues to advance and as users become more accustomed to using chat-based AI tools in their everyday work, the flaws in the answers are becoming more difficult to spot. Allison Kostecka commented, “Don’t just ask one question, ask five. Compare the answers and validate them in different ways.”
Kostecka also commented that trust begins in the early stages of evaluating AI technology. It starts with being able to test tools in a secure way to ensure they do what they’re supposed to do. Kostecka suggested that, when testing a new AI tool, it is best practice to compile a benchmark dataset that can be ingested into the tool and interrogated to validate the tool’s capabilities and output. Kostecka’s team has compiled benchmark data sets using publicly available data related to the litigation at hand.
The journey towards trustworthy AI will always be an ongoing pursuit. Continuous advancements in AI technologies must be accompanied by ongoing efforts to enhance transparency, accountability, and ethical standards. As Dooley summarised, “Trust is not just about the technology itself; it’s about the entire ecosystem that supports it, including the legal and ethical frameworks that govern its use.”
Learn more about Epiq AI applications and the range of legal use cases they support, including Knowledge Management, Deposition and Trial Preparation, Contract Lifecycle Management, and Discovery.
The contents of this article are intended to convey general information only and not to provide legal advice or opinions.