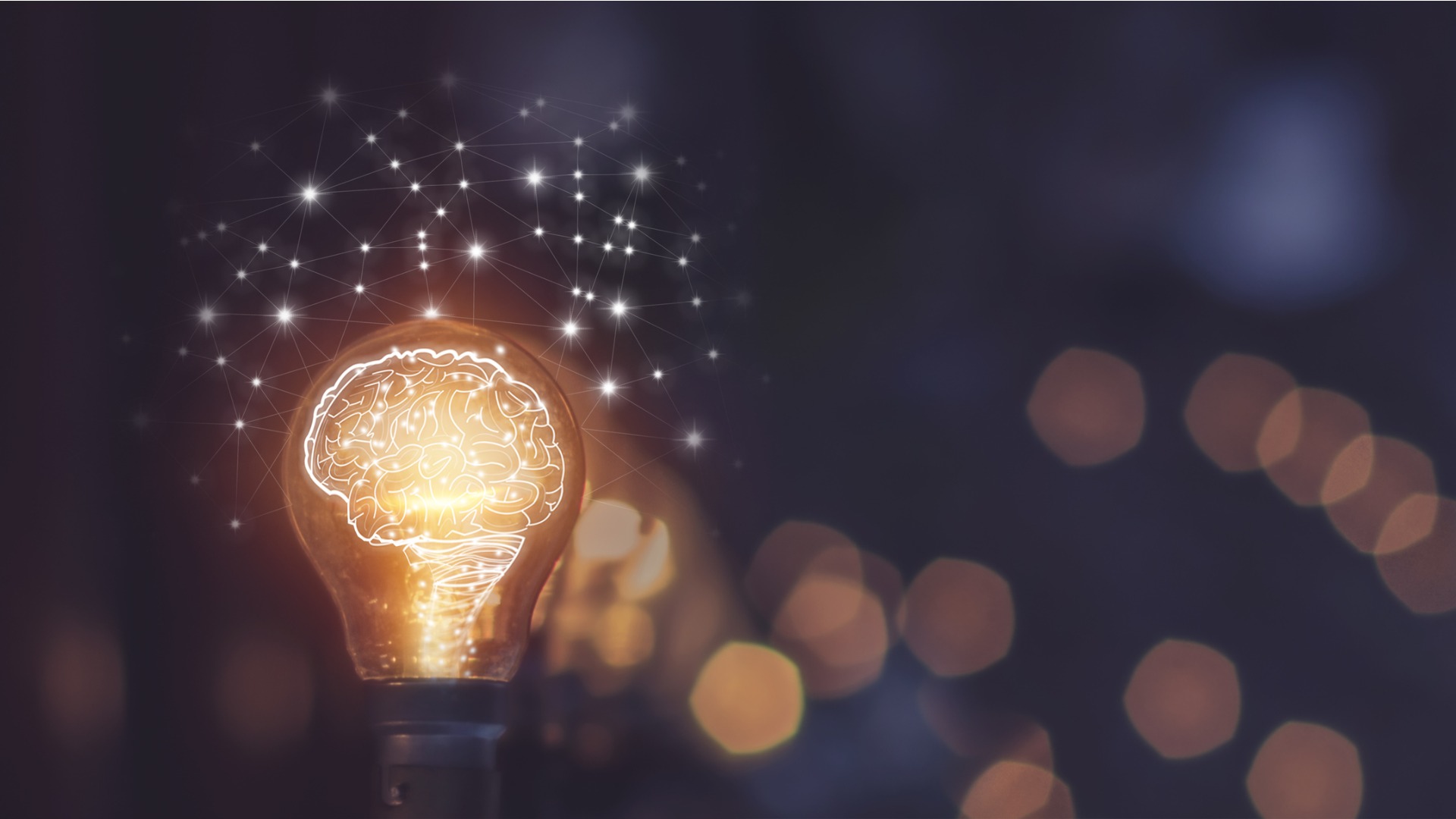
Article
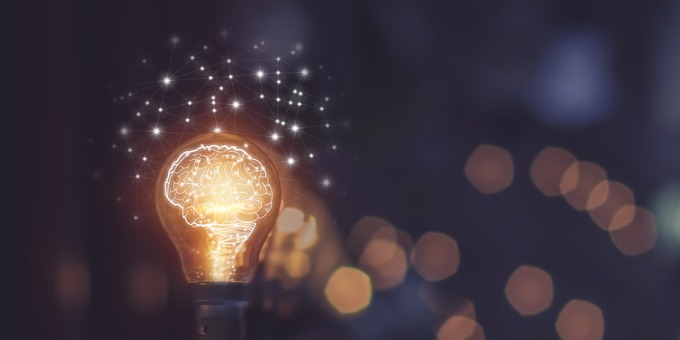
AI Evolution: Prompting and Problem Solving
- eDiscovery
- 3 mins
The world of artificial intelligence (AI) is evolving at rapid speed, especially with the rise of generative AI tools. Large language models (LLMs) like ChatGPT have the potential to automate or expedite any task that requires the recognition and generation of textual content at quality levels that are often indistinguishable from human-generated text. These LLMs are in the early stages, so the output often lacks context and requires human review. This will improve quickly as the model trains with more data.
In the legal industry, the prospective generative AI use cases for both corporate legal departments and law firms are plentiful. The most significant short-term opportunities will likely be optimizing internal processes. Examples include generating reminders around security, compliance, and information summarization across commercial contracts; incorporating generative AI into existing eDiscovery solutions; template creation; and brief drafting.
As with any new technology, the implications of usings LLMs are a top concern in the legal industry. There is a desire for deeper understanding of how this technology works to determine optimal use cases and limit risk. Prompt engineering and problem formulation are two areas to explore further, as these processes are paving the way for better-trained models.
Prompt Engineering
Prompt engineering refers to the process of understanding and refining the questions a user asks an AI or LLM system to get optimal results. The ability to optimize questions lead to better output with minimal amount of back-and-forth prompting. Users have found that merely asking questions without being strategic can lead to generic or incorrect output. Industry leaders in this area are helping to formulate these prompts, which will prove valuable and limit risk in the legal use cases noted earlier.
Best practices have surfaced regarding how to strategically ask questions. This includes prompting the tools to tell the user what else it needs to do a certain task or solve an issue; apply a specific framework to a problem; or act as if it is a person in a certain profession. Prompts like this help direct LLMs to the right data, output more personalized results, and be refined over time through conversation threading. For example, using the prompt “act as if you are a tutor for the Bar exam” would guide the tool to training data specifically from this area. Having more context allows the bots to generate more tailored responses and lessens the risk of receiving false information.
The quick evolution of AI models leads to less prompting needed over time. This will only continue and allow systems to learn at a faster rate. As advancement occurs, these systems may even be able to craft their own prompts. Linguistical challenges may also arise, as prompt engineering requires a strong focus on the language used to craft questions. Even a small linguistical nuance can alter the output. Some industry professionals believe that the need for prompt engineering is not as significant as first understood because of these reasons. For now, having a partner that is at the forefront of prompt engineering can help organizations use these tools responsibly and open the door for focus on more specialized needs such as problem formulation.
Problem Formulation
Problem formulation is a skill that some analysts believe is the real area of need when it comes to harnessing and training AI systems. This requires getting a firm grasp on the problem needing to be solved in order to pinpoint the right input. This process differs from prompt engineering, which focuses on the capabilities of a specific tool to determine the best questions to ask.
Along with prompt engineering, problem formulation is a developing area. To hone this skill, several competencies become important. This includes being able to diagnose a problem succinctly, breaking down complex problems, reframing issues, and thinking of the constraints needed to direct an AI system. With complex legal issues, this requires the access to the right expertise and technologies.
Being able to clearly define a problem should allow users to guide these tools better, alleviate linguistical roadblocks inherent in prompting, and maintain creativity and control when formulating a solution. If the problem is clearly defined, then any issues with the language used in a prompt will no longer act as barriers to reaching the solution. This aligns with goals inherent in legal practice – getting clients the best results in the most efficient manner while still maintaining legal judgment over the ultimate solution. It will be interesting to see if and how the “problem formulation approach” trends.
Conclusion
For now, it is important to monitor the developments with both prompt engineering and problem formulation. Even if focusing on the problem becomes more mainstream, prompts will remain a valuable asset to have in order to use AI tools more effectively. These two processes will likely intertwine in the future. Having a partner that is a pioneer in these areas will allow corporate legal departments and law firms to decide on appropriate use cases, be strategic, safely use these tools, and maintain marketability.
The contents of this article are intended to convey general information only and not to provide legal advice or opinions.