
Advice
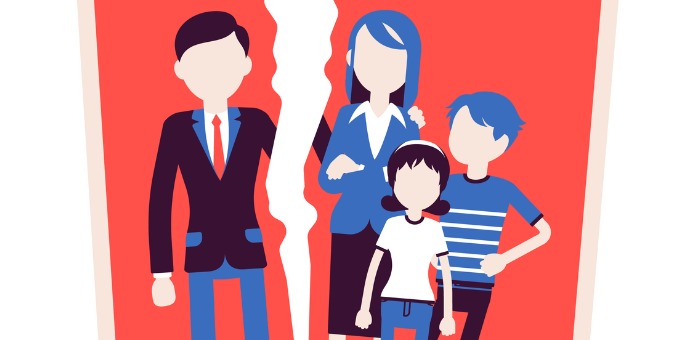
Three Benefits of Breaking Up Document Families for Review
- eDiscovery
- 3 Mins
It is a safe bet that every litigator has either used technology-assisted review (TAR) in practice or is at least familiar with this technology. TAR solutions use machine learning algorithms to classify large volumes of electronically stored documents. TAR can help lawyers reduce the time and cost of reviewing documents, as well as improve the accuracy and consistency of their decisions. It has been around for years and best practices keep evolving as this technology matures.
One key area to consider is how to review documents that are part of a family. Not all documents are created equal, some may need to be produced in families to comply with discovery requests. These document families generally share common characteristics such as metadata, content, or relevance. Reviewing document families together may seem like a logical and efficient practice, but it can introduce challenges and risks while protracting the timeline with unnecessary review.
Here are three reasons why legal teams should consider breaking up document families and performing separate reviews in a TAR workflow:
#1: Avoiding Bias and Contamination
Reviewing document families together can create bias and contamination in the TAR model. Information in one document may inform the coding of a family member, something that reviewers must avoid to achieve a quality TAR model. Breaking up these families can help avoid these problems by ensuring that each document is evaluated independently based on its own merits. This is often referred to as the “Four Corners Rule”.
#2: Increasing Efficiency and Quality
Reviewing documents separately can increase the efficiency and quality of the review process. TAR 2.0 workflows can sort and prioritize documents as reviewers code them, constantly funneling the most responsive ones to the top for review. By only reviewing documents likely to be responsive, the solution can uncover a larger number of relevant documents more quickly. Additionally, because not every document prioritized for review will be responsive, breaking up the families eliminates unnecessary and costly review of wholly non-responsive families. Breaking up document families can help leverage TAR tools and workflows more effectively by reducing noise and increasing signal in the dataset, resulting in a better and more efficient TAR model.
#3: Increasing Speed and Maintaining Recall:
According to a 2018 study by Big Data, breaking up document families resulted in faster review speed and higher review accuracy compared to reviewing them together. The study also found that breaking up document families did not significantly affect recall, which refers to the percentage of relevant documents identified (Jeremy Pickens, Thomas C. Gricks, Esq., Andrew Bye, Break up the Family: Protocols for Efficient Recall-Oriented Retrieval Under Legally-Necessitated Dual Constraints, 2018 IEEE Int’l Conf. on Big Data (Big Data), 3302).
Furthermore, industry research does not support the notion that a linear review represents a gold standard for recall. A 2005 study by Anne Kershaw found that the computerized system identified 95 percent of relevant documents, while a team of humans only identified 51 percent Anne Kershaw, Automated Document Review Proves Its Reliability. http://www.akershaw.com/Documents/2004AEKDocReviewArticle.pdf Accessed 3/20/2023). Other research suggests that the best human reviewers could hope for was 65 percent precision at 65 percent recall, as that is the rate at which humans agree with one another (See, e.g., Maura R. Grossman & Gordon V. Cormack, Technology-Assisted Review in E-Discovery Can Be More Effective and More Efficient Than Exhaustive Manual Review, XVII RICH. J.L. & TECH. 11 (2011), at 10-15 (summarizing recent research on human review and citing results for a maximum of 65% recall (Voorhees 2000) and 52.8% – 83.6% recall (Roitblat, Kershaw, & Oot 2010)).
In summary, breaking up document families has emerged as a best practice for TAR workflows in eDiscovery. It will help avoid bias and contamination in the TAR model; increase efficiency and quality in the review process; and ultimately save time and money for lawyers and clients. On top of this, it remains at least as effective as a full human review and is often even more accurate. Legal teams should consider monitoring best practices in the TAR space, as with eDiscovery in general, as new legal tech and creative solutions enter the industry.
By: Stephen Tryon, who is an Advanced Technologies Consultant in the Epiq Salt Lake City office. He has five years of experience using advanced analytics and building artificial intelligence models to facilitate more efficient eDiscovery reviews. Stephen has worked with top law firms and companies across the globe to develop workflows and solutions to the challenges presented by an ever-growing data economy, including consulting on consumer-facing AI software offerings.
The contents of this article are intended to convey general information only and not to provide legal advice or opinions.