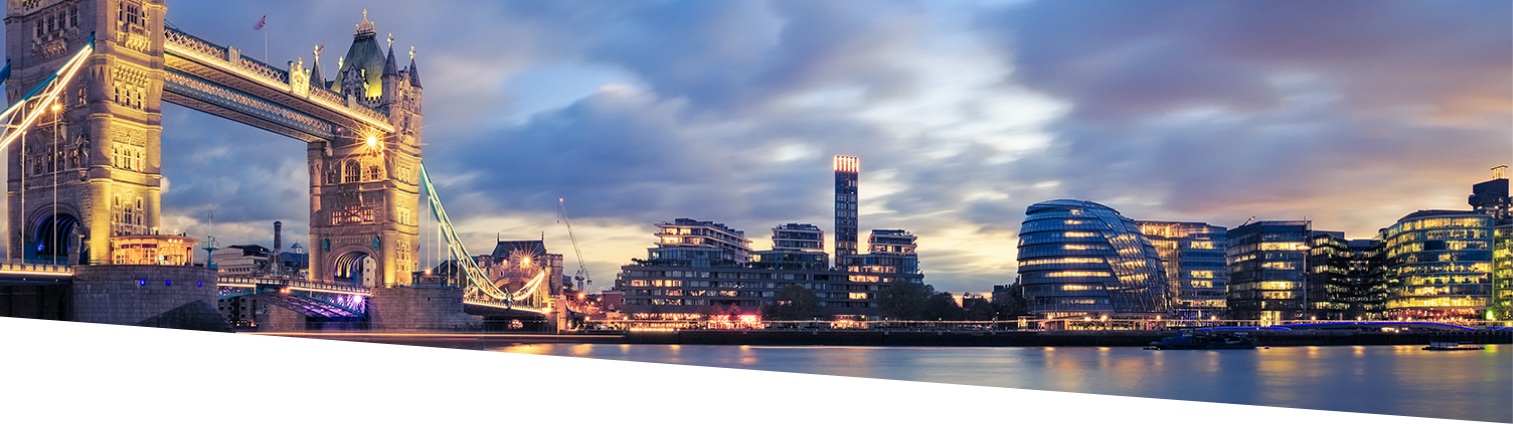
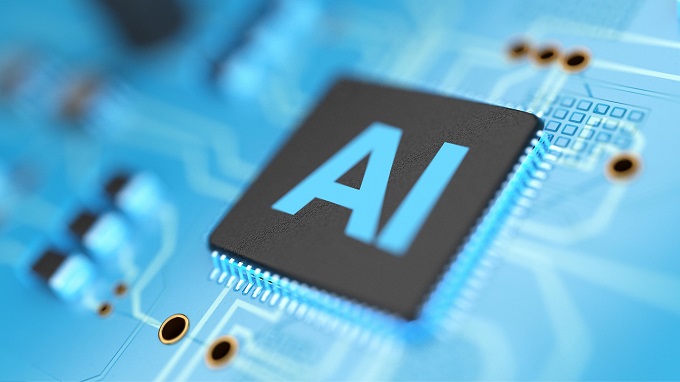
Artificial Intelligence: Using Advanced Analytics to Detect Conduct and Patterns of Behavior
- eDiscovery and Investigations
- 4 mins
Artificial intelligence (AI) adoption has been largely accepted in the legal community, as many have realized the value of technology that can detect relevant content and produce better outcomes. Incorporating AI into document review workflows or using insights to inform case strategy is transformative and drives better results. From government requests to civil litigation and internal investigations, high profile and fast-moving matters require efficient processes. Deploying technology strategically will help teams to identity key documents and themes early in the case and manage the assessment and review of data efficiently. The continued evolution of AI tools, such as the ability to detect conduct and behavior through sentiment analysis and pattern processing, will further assist with investigatory compliance but can also be used proactively.
Growing Acceptance of AI in the Legal Community
There was a time when skepticism clouded the potential value of predictive models emerging over the eDiscovery horizon. Lawyers were quick to refute the possibility that machine learning and classification of documents could potentially offer a defensible solution to increasing discovery volumes. In a field characteristically resistant to change, this was expected. Despite widespread adversity, advocates such as Maura Grossman and Judge Andrew Peck noted the success of predictive modeling and, as thought leaders in the eDiscovery space, initiated gradual acceptance. The analytics naysayers now appear to represent the minority and the use of predictive models is widespread in Technology Assisted Review.
Yet even during the early days of evolution, when the legal community was just starting to become familiar with TAR protocols and analytics tools, the use of predictive models was already expanding. Advanced investigatory features such as pattern processing and sentiment analysis were introduced as newcomers to the eDiscovery analytics market despite longevity in the field of data science. As use became more widespread, law firm associates and in-house counsel were often impressed during demos, but seemingly hesitant to embrace the functionality within a live workflow. Clients started asking how to effectively leverage these tools and requested assistance with workflow implementation. Market demand necessitated a process devoted to using these tools and legal technologists rose to the challenge.
Exposure to vast amounts of data, with dramatically different content, enabled well-versed users to synthesize the results of both sentiment analysis and pattern processing and develop applicable use cases. It became evident that text-based communications amidst unstructured data yielded the most valuable results, particularly when used as part of investigations or early case assessment. Since “sentiment analysis tools attempt to automatically label the subjective emotions or viewpoints expressed by text,” it follows that email communications would be a target rich data pool for this functionality. See John Nay, Natural Language Processing and Machine Learning for Law and Policy Texts (New York University April 7, 2018). In model form, sentiment analysis can be searched upon by score and incorporated as an element of searching. In doing so, when interrogating data, we have an intelligent way to prioritize the search results of custodian Jane Doe, from year 2015, that hit on search terms X, Y and Z. We can sort those results based on sentiment score. Much to the dismay of discovery attorneys, it is still necessary to set eyes on documents to validate the results (and perhaps perform secondary coding); but in theory, users will uncover the most emotionally charged results at the onset of investigation, as opposed to after a full linear review. The logic behind this method is also applicable to pattern recognition. For example, if we consider the above-referenced custodial data example again, the most anomalous results can be identified and displayed first. This means that if Jane Doe logged off her computer at 5:00 pm every day during her five-year tenure with A Corp, and then suddenly started sending email communications at midnight for a whole week in January, the analytics tool would likely identify this pattern as an anomaly. This can be a valuable function that produces high value results with a relatively low effort on the part of those investigating.
When used carefully, these features offer powerful techniques to identify unusual behavior, potentially inappropriate conduct and emotional exchanges, which are often tied to the underlying legal issues in internal investigations and litigation. Specific examples of conduct that are ripe for discovery using these tools include cartel activity, such as price-fixing and other anti-competitive behavior, sexual harassment and inappropriate relationships, and collusion and employee misconduct. However, potential use cases should not be limited to these situations.
This blog post is an excerpt from the Chapter titled “Outsourced Document Review: Data Intelligence, Technologist Lawyers, Advocacy Support” by Edward Burke and Allison Dunham, which appears in the Thomson Reuters treatise eDiscovery for Corporate Counsel (2022). Reprinted with permission, © 2022, Thomson Reuters. Ed and Allison are eDiscovery experts at Epiq.
A link to the book appears below:
https://store.legal.thomsonreuters.com/law-products/Treatises/eDiscovery-for-Corporate-Counsel-2022-ed/p/106770308
The contents of this article are intended to convey general information only and not to provide legal advice or opinions.